The Essential Guide to Video Annotation Tool for Computer Vision
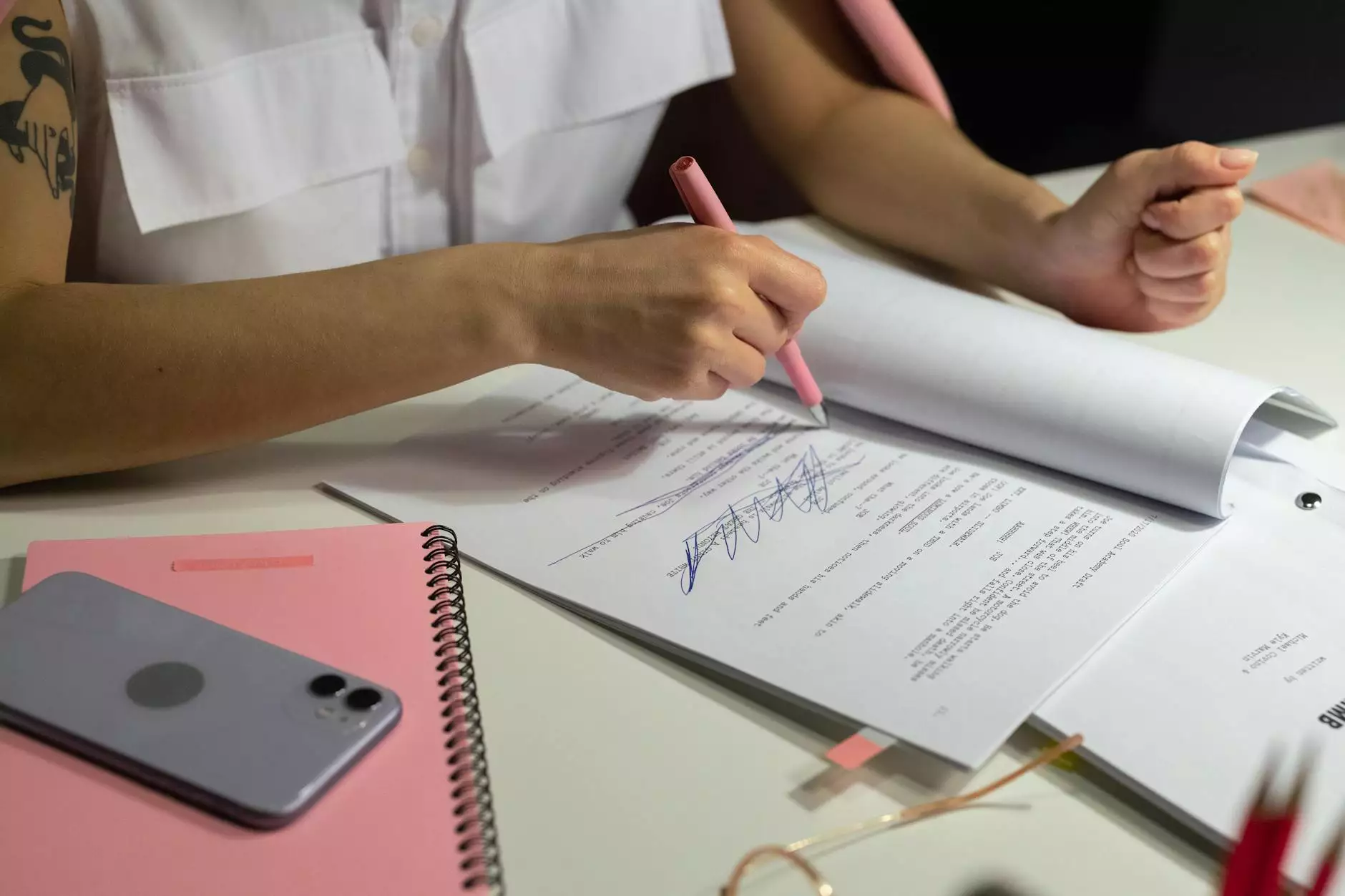
The rise of artificial intelligence (AI) and machine learning (ML) has led to unparalleled advancements in various sectors, particularly in computer vision. Central to these developments is the need for accurate and efficient data processing, especially in understanding visual information. One of the most vital components of this process is the video annotation tool computer vision, which facilitates the annotation of video data essential for training machine learning models.
What is Video Annotation?
Video annotation is the process of marking or labeling specific segments or objects in video footage. This process is crucial for training AI models to recognize patterns, objects, and actions within videos. For instance, in the context of autonomous vehicles, highlighting important features like lanes, pedestrians, and traffic signs in video feeds enables vehicles to make accurate driving decisions.
Why Use a Video Annotation Tool?
Utilizing a dedicated video annotation tool for computer vision provides several advantages for businesses and developers:
- Improved Accuracy: Automated tools enhance the precision of annotations, reducing human error.
- Efficiency: These tools streamline the annotation workflow, saving valuable time for data scientists.
- Scalability: They allow for rapid processing of large datasets, essential for machine learning applications.
- Versatility: Support for various annotation types, including object detection, action recognition, and semantic segmentation.
How Does a Video Annotation Tool Work?
A typical video annotation tool functions through a series of steps that ensure video data is accurately labeled:
1. Uploading Video Data
The first step involves uploading video files to the annotation platform. Most tools support common formats like MP4, AVI, or MOV, allowing easy integration of existing projects.
2. Selecting Annotation Types
Users can choose from various annotation types depending on their project needs, including:
- Object detection (bounding boxes)
- Semantic segmentation (pixel-wise labeling)
- Instance segmentation
- Action recognition (event labeling)
3. Annotating the Video
Once the type is selected, users can start annotating. This process typically involves marking objects or actions in each frame of the video. Advanced tools often include functionalities for:
- Frame-by-frame navigation
- Time-stamping annotations
- Collaborative features for multiple users
4. Exporting Annotated Data
After completing the annotation, users can export the annotated data in various formats (like JSON, CSV, or XML) that are compatible with machine learning frameworks such as TensorFlow or PyTorch, ensuring seamless integration into AI workflows.
Key Benefits of Using Video Annotation Tools
In the competitive landscape of AI and machine learning, leveraging a video annotation tool for computer vision can confer numerous benefits, such as:
1. Enhanced Model Training
Annotated video data leads to more robust training of machine learning models, enabling them to generalize better to real-world scenarios.
2. Faster Turnaround Times
Automating the annotation process significantly decreases the time required to prepare datasets, allowing teams to focus on development rather than tedious data preparation tasks.
3. Cost-Effectiveness
Investing in a reliable annotation tool can reduce labor costs associated with manual annotation efforts and lead to faster project completion—ultimately saving money for businesses.
Choosing the Right Video Annotation Tool
Selecting a suitable video annotation tool computer vision requires careful consideration of specific features and capabilities. Here are key factors to evaluate:
1. User Interface and Experience
The tool should have an intuitive interface that simplifies the annotation process. Easy navigation and functionality can significantly affect productivity.
2. Collaboration Features
For teams working on large projects, a platform that supports real-time collaboration is essential. This allows multiple contributors to work simultaneously, enhancing efficiency.
3. Compatibility with Machine Learning Frameworks
Ensure the tool can export data in formats that integrate seamlessly with your existing machine learning pipelines. Compatibility with popular frameworks such as TensorFlow, PyTorch, and OpenCV is a significant plus.
4. Customization Options
The ability to customize annotation types and workflows based on your project requirements is crucial, particularly for unique applications such as medical imaging or video surveillance.
5. Quality of Support and Documentation
Access to excellent customer support and comprehensive documentation can make a significant difference, especially when onboarding new users or troubleshooting issues.
Applications of Video Annotation in Various Industries
The versatility of video annotation tools allows for their application across numerous industries:
1. Automotive Industry
In the automotive sector, annotated video data is used extensively for developing self-driving technology, enabling vehicles to navigate safely by recognizing objects and predicting actions.
2. Healthcare
Video annotation is increasingly utilized in the healthcare sector for analyzing patient data, such as identifying anomalies in surgical procedures or monitoring patient movements for rehabilitation purposes.
3. Retail and Surveillance
Retailers use video annotation to analyze customer behavior, optimize store layout, and improve inventory management. Surveillance systems employ these tools to identify and track individuals or vehicles in real-time.
4. Sports Analytics
Sports organizations harness video annotation for performance analysis, enabling coaches to dissect gameplay and strategize effectively based on player movements and actions.
Future Trends in Video Annotation
As the demand for annotated data continues to rise, several trends are emerging in the realm of video annotation tools:
1. AI-Powered Automation
Artificial intelligence is set to revolutionize video annotation through automation, reducing the need for manual labor while increasing accuracy. Automated systems can learn from existing annotations and suggest labels, dramatically speeding up the process.
2. Advanced Collaboration Features
Future annotation tools will likely include enhanced collaboration tools that allow for seamless communication between team members, further enhancing productivity and project outcomes.
3. Integration with Augmented and Virtual Reality
As AR and VR technologies grow, the need for accurate video annotation in these environments will push the demand for more sophisticated tools capable of handling complex datasets.
4. Increased Customization and Flexibility
Businesses will demand more tailored solutions that allow them to configure annotation environments according to their workflows and requirements, leading to greater adoption and specialization.
Conclusion
In summary, the significance of a video annotation tool computer vision cannot be overstated. It is a fundamental resource for organizations aiming to leverage the power of AI and machine learning. By selecting the right tools and implementing efficient annotation processes, businesses can kickstart their journey into the data-driven future, equipped to compete and innovate effectively.
Whether your organization is stepping into the world of AI or looking to enhance existing capabilities, investing in a video annotation solution is a crucial step toward achieving your goals. Platforms like KeyLabs.ai offer comprehensive options that cater to diverse needs in data annotation, ensuring you remain at the forefront of technological advancements.